French National Research Agency – Generic Projects 2022
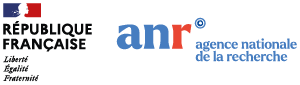
Integrating multiple sources of evidence for optimized comparative effectiveness research (OptiCER)
Principal Investigator: Anna Chaimani
Participants: Tianqi Yu, Theodoros Evrenoglou, Raphaël Porcher, Isabelle Boutron
Host Institution: METHODS Team, CRESS
The systematic identification and synthesis of all available evidence on a specific clinical question is an integral part of the medical decision-making process and the planning of future research. For most medical conditions, a plethora of healthcare interventions are available and, thus, network meta-analysis (NMA) – the only tool enabling simultaneous comparisons between many interventions – is currently among the highest levels of evidence for the development of clinical guidelines. However, current NMA methodology has important limitations for properly synthesizing different study designs (randomised, cohort, case-control, etc.), which could increase the external validity of the findings. These limitations impose restrictions in most NMAs by usually allowing the synthesis of a selective sample of randomised controlled trials only, rendering their results rarely applicable in real settings of healthcare practice. The aim of the OptiCER project is a) to develop and evaluate new synthesis methods for integrating all different sources of evidence available for addressing a common research question and b) to develop new presentation and communication tools for presenting the findings of these ‘all-evidence’ NMAs targeting the needs of different stakeholders (clinicians, regulators, guideline developers). All new statistical models and tools will be accompanied by open-access and user-friendly software. The new methods will be used for the synthesis of the COVID-19 studies but will also be freely available for application in other medical fields. In this way, clinical recommendations will be based on a global view and synthesis of any piece of evidence rather than on a partial approach.
Other projects we participate
Completed projects
Marie Skłodowska-Curie Individual Fellowship – Dynamic Comparative Effectiveness Research for health care interventions (DyCER)
French National Research Agency – AAP Flash Covid-19 – Interventions for Covid-19 infection: a living mapping of research and a living network meta-analysis
Assistance Publique – Hôpitaux de Paris (Clinical Research and Development Department) – Live cumulative network meta–analysis: Systemic pharmacological treatments for chronic plaque Psoriasis
Principal Investigator: Emilie Sbidian
IdEx Université Paris 2019 Fellowship – Dealing with sparse data in network meta-analysis
Université Sorbonne Paris Cité Chair of Excellence Fellowship – Methods for Evidence Synthesis
Principal Investigator: Anna Chaimani
Host Institution: METHODS Team, CRESS
Washington University Institute of Clinical and Translational Sciences – Career Development Awards Program
Principal Investigator: Sonal Patil